Optimizing Learning Through The Use Of Learning Analytics
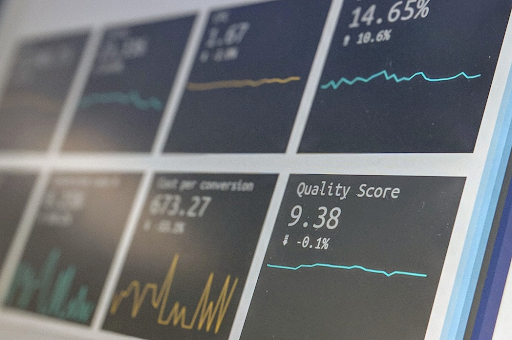
Since the beginning of the 2010s and even more so in the wake of the current COVID-19 pandemic, learning has been digitized and learning environments have been digitized. This growing development is resulting in a “boom” of data collected from different learning platforms. Thus, the current interest in learning analytics is just a reflection of what Big Data is also experiencing. Big Data is characterized by three factors: volume, velocity and variety. It thus allows us to understand the uses and practices of individuals but also to design theoretical and behavioral models and predict trends. In this way, Big Data fits both in the immediacy by having real-time access to the needs and habits of individuals and in the long term for the creation of predictive models.
The reasons for the continued interest in learning analytics lie primarily in the massive amount of education data, the continued increase in processing power, and the motivation to deduce new insights from the available data.
Learning analytics or digital learning analytics in French, are defined, according to Siemens and Baker 2012, echoed by Fischer, Hmelo-Silver, Goldman and Reimann in 2018, as “the measurement, collection, analysis and reporting of data about learners and their contexts, for purposes of understanding and optimizing learning and the environments in which it occurs“. More simply, it is a tool for understanding learning processes and context that enables discovery and prediction. It can also be used for the very improvement of processes in digital environments.
This emerging economy is just the emerging part of Big Data and this one is of particular interest to companies and large commercial groups that are looking to better understand the habits and practices of learners as consumers to better target and optimize their commercial offerings and of course predict future trends. According to a study conducted by the Center for Digital Education with higher education faculty, the main benefits of Big Data analytics in education would be tracking and predicting a student’s performance (69%), increasing graduation rates (61%), making real-time adjustments to curricula (47%), measuring institutional performance of the institution (44%), and uncovering potential flaws in the administration through analytics (22%).
This is a set of issues that must be addressed now if they are not to be caught up in the speed of technological development and overwhelmed by events.
The learning analytic sare computer, mathematical and statistical techniques for detecting relevant information from a massive set of data. Therefore, the term “analytics” should be interpreted as an action performed with the objective of understanding and even predicting future actions in pursuit of ever greater performance and efficiency. This method is significantly closer to data science (Data Science) and thus meets the principle of Data Mining.
The subject matter of learning analytics differs from the previous two scientific methods in that it is multidisciplinary and works toward the design of computational environments conducive to human learning.
The reasons why scientists are particularly interested in learning analytics are:
– The enormous volume of data available to researchers that allows for rapid progress and the proliferation of online learning devices such as the Learning Management System, MOOCs). All of them enable the isolation of user interactions during online learning. It is also possible to cross-reference this data with academic data.
– Structuring this data allows for rapid use and therefore more interoperability for researchers.
– The devices used, today, to take online lessons, such as computers, smartphones, or tablets, have extremely large computational capabilities.
– Computer programming (framework), including Apache Hadoop, Rapid Miner, KEEL, SNAPP, allow for management and measurement of data from the Internet. Indeed, many analytical tools are gradually being adapted to education such as Business intelligence without prior knowledge of statistical science.
Learning analytics allow the data produced during learning activities to be tracked. As such, said data can be of a conventional nature i.e. from exam results, participation rate during activities and more generally attendance rate, end of year exams and diplomas obtained. These data are traditionally collected by teachers face-to-face. But progressively, the new digital environments are also places where data are collected, as is the case for learning platforms and personal spaces on the intranet. These new ecosystems provide considerable bodies of data on the behavior of learners ranging from the connection, to the various fingerprints deposited by the latter and which are automatically stored by the computer server before being processed by algorithms capable of analyzing and establishing the user’s profile.
All these reasons make it all the more necessary to recall the indispensable characteristics that learning analytics must meet, which are:
– Capitalization and continuous collection because the data collected is the raw material of the process.
– The multiplication and multi-disciplinarity of the sources of origin of the data (face-to-face, remote, continuous from the beginning of the educational pathway).
– The phenomenal amount of data produced by learners through the use of digital tools in learning environments.
– The specificity because the data collected is only about the activity of learners in order to improve the learning experience and learning environments.
Subsequent to these different characteristics, three major functions can be attached to learning analytics i.e. the descriptive function, the predictive function and the prescriptive function. Each of them helps to explain the surpassing of analysis allowed by digital learning analytics compared to the classical field of educational sciences.
The descriptive function focuses on describing the particular context in which learners operate. This extends from the conditions to the expectations of learners themselves during their learning while taking into consideration the courses, the resources available and the tasks to be performed in the digital environment. In other words, it is a matter of evoking the characteristics of learning, the learning strategies adopted while knowing that they can be varied.
In this analysis, three elements are paramount in predicting success, failure, or dropout of learners (one of the goals of learning analytics):
– Characteristics of the teaching environment and specifically how learning is delivered to learners.
– The characteristics intrinsically related to the learners.
– The interactions between the teaching environment and the learning strategies used by the learners.
Beyond a simple analysis, this is a real study of the links between the three elements mentioned above, which should allow the realization of typical “profiles” to which teachers can refer later on in order to recognize the situations in which each student finds themselves.
The predictive function, on the other hand, focuses on trends determined from the learners’ context and situations. Subsequently, they will be nuanced by machine learning. With this system continuously fed with new data, it is possible to influence and predict the success of learners.
The prescriptive function corresponds to the expected reaction to the situation described by the two previous functions. In other words, it focuses on proposing individual recommendations that can support, accompany and guide learners on the objectives they had originally set for themselves. The advice offered must be based on past experience and be sufficiently reliable.
With real-time access to learning data, researchers have developed analytic techniques, which are significantly closer to Data Mining, making it possible to classify profiles. These classifications take into consideration learner ability, content adaptability, level of deployment of engagement strategies, and methods to combat dropout.
Social Learning Analytics are based on the analysis of social networks and existing communities within them, the subsets created by these networks but also their proximity, the frequency of exchanges, their density and the affinities that emerge. From these observations, it is possible to identify accompanying persons and thus to characterize the links between the different actors.
The study of social networks presents a new and vast scope for deepening social learning. They allow us to considerably broaden the techniques of measurement and representation of biometric data thanks to the recurrent use of cameras that allow us to examine the synchronization of physical postures. Following this line, it is quite relevant to imagine that in future it will be possible to analyze new indicators such as voice or heart rate to measure the intensity of social interactions (GTnum2).
Language influences, in part, the success of activities, the use of tools and the success of joint work. This is why discourse analysis is an element to be taken into account by learning analytics because it makes visible and therefore allows for the improvement of the individual’s place within the group.
Learning analytics must be perceived as tools at the service and in support of the regulation and self-regulation of learning. This necessarily induces awareness from the information made available, the questioning of the learner, the adaptability of learning strategies, the production of avenues for regulation and improvement. Learning analytics have a wide variety of assets ranging from the objectivity of the elements (decisions, results and behaviors) to the speed and regularity of feedback available to learners and teachers.
Learning analytics are the result of a cycle of traces passing through different stages such as collection and processing. It is undeniable that said steps raise issues of personal data protection intimately related to privacy. Even data that appears to be ” harmless ” can affect the privacy of individuals. Moreover, consent to the collection of data, to the processing and to its reuse by online services for specific, distinct purposes presented to the user only in rare cases allows control over the information that will potentially be produced by the automated aggregation of applications present on websites, applications, etc.
The General Data Protection Regulation (GDPR) that was adopted in 2016 and came into force on May 25, 2018 tends to slow down the development of learning analytics. All processing, undertaken by the software using learning analytics must be lawful and justified. The context has a very great importance because depending on it, the controller and/or its processor will have to justify the lawfulness of the processing.
Today, data protection is an integral part of the learning analytics research approach.
In the case of a learning analytics development project, the specifics of each institutional project will need to be considered. In most cases, the approach will as a minimum be confronted with GDPR in two cases such as large-scale data collection, data cross-referencing or innovative use of said data. This is due to the large number of learners that allow the creation of personalized interfaces, the creation of typical profiles. It is important to know that digital learning analytics also process administrative data (enrollment, etc.), performance data (exam results, etc.), and usage data of learning resources offered by the institution in question. In addition to this, it is rare that the data is stored on a single database. .
For all these reasons, it is essential to conduct an impact analysis upstream and submit it to the institution’s data protection officer for approval.
It appears that data exploitation is central to the digital learning analytics approach. Data is therefore a major issue for this emerging discipline. The objective is to improve the quality of learning without increasing the workload of teachers and other supervisory staff. Maximum automation is also an issue in the data processing process because it allows for rapid decision-making by the learning actors. Of course, automation must also be GDPR compliant.
On top of this first regulatory layer, some researchers have proposed the addition of the ” DELICATE Checklist ” model to allow learning analytics practitioners a reflection on privacy aspects. More simply the idea is to continue to put human beings at the center of concern while being transparent and open. This model has not yet been implemented.
Even if the purpose of learning analytics is rather benevolent in relation to the learner, their conditions of use and exercise can raise many questions.
In fact, despite the principles derived from GDPR, these are, with regard to learning analytics, limitations but in reality it is difficult to determine in advance what information about the environment and the results are likely to be relevant to the study. Therefore, the very activity of learning analytics is part of an exploratory approach that allows for retrospective modeling. This means that digital learning analytics does not seek to validate theoretical hypotheses but rather conducts research to hypothesize online learning habits. The viewpoint is completely contrary to what is done in the humanities and social sciences. It’s also hardly compatible with the principle of minimizing data collected under GDPR. Hence why this practice is so criticized.
To further explore the issue of minimizing collected data, many questions need to be asked such as the relevance of the data, is it limited to learning data? Is it school data only or does it also cover social (scholarship student or employee, etc.) or even psychological areas? (GTnum2).
In addition to this first difficulty, there is the lack of an official definition for school data. Thus the perimeter covered by this type of data is particularly wide, ranging from administrative information, to productions rendered, to learning traces. This explains why it is fundamental to organize a real ethical reflection on data collection. The relevance of the data is measured in terms of the purpose pursued.
Another danger that has been somewhat addressed is the combined use of data on learners’ learning with data on their bodily privacy (fingerprints or facial recognition, etc.). The widespread surveillance of the body through learning analytics is a real problem especially for people entering higher education while still minors. But in addition to this specificity, the collection of physiological data (like the Knewton example) early and from people who are likely to forget that this data was ever collected is a risk that should be anticipated. The right to be forgotten should not be omitted, hence the importance for learners to be aware and conscious of the data collected.
Another important aspect that has not been highlighted at all is the possibility offered by learning analytics to preserve anonymity or at least contribute to data privacy. Anonymization is a big challenge because with data cross-referencing, it is very easy to identify people without having their names.
It is impossible to contradict this obvious fact: learning analytics are a form of permanent surveillance during courses or exams. Indeed, since it is possible to know, for each learner, when they left the course, if they re-listened several times to a part of the course etc… Similarly, the use of the camera allows facial recognition (artificial intelligence) and therefore the collection of physiological data but also to record the emotions of the learner. This again refers to the importance of consent and awareness of learners about the collection of their data.
Without going back to the specific case of minors, is consent in the educational setting truly free? Indeed, it is quite legitimate to wonder whether, in the educational framework, the student can be considered as validly giving his consent with regard to the authority exercised over him by the teacher who proposes the use of such a tool? As a reminder, Article 4 of GDPR states that consent constitutes “any freely given, specific, informed and unambiguous indication of the data subject’s wishes by which he or she, by a statement or by a clear affirmative action, signifies agreement to the processing of personal data relating to him or her;.” Is this really respected when there is a form of authority weighing on the signatory, i.e. the learner who is going to be evaluated by the said teacher?
Notwithstanding this possible existing “pressure” to consent to the collection of data in order to use the tool submitted by the teacher, another question arises, that of knowing whether the learning is part of a public interest mission?
Learning analytics are used to obtain information about the learners’ learning and thus allow them to be ranked against each other. Thus, learners are quantified or rather their personality is measured in order to achieve the best performance. In other words, learning analytics undermines the very essence of the learner’s personality. In fact, by associating learning platforms with sensors in order to analyze ever more finely the reactions of learners and to offer support as close as possible to the needs of each individual in order to implement personalized learning.
The expected benefits face the phenomenon of the “quantified self” which was studied in 2013 by the CNIL in its study “Le corps, nouvel object connecté”. Through the quantification of learners’ performance, is it possible that learners develop a form of compulsory, anxiety-provoking normalism? Similarly in the opposite case, isn’t the search for personalization of learning with the aim of improving the learner’s performance likely to turn into an obsession? Especially since success is not only reflected in results and grades.
In any case, learning analytics must not transform learners into learning “objects”; they must remain a service for the actors. In other words, the massification of analyzed data and objectification should not contribute to the emergence of a new discipline. In the same idea, the learner should not tend to become a simple passive producer of data; the relevance of the data should remain a priority.
GDPR and Compliance
Outsourced DPO & Representation
Training & Support
To give you 100% control over the design, together with Webflow project, you also get the Figma file. After the purchase, simply send us an email to and we will e happy to forward you the Figma file.
Yes, we know... it's easy to say it, but that's the fact. We did put a lot of thought into the template. Trend Trail was designed by an award-winning designer. Layouts you will find in our template are custom made to fit the industry after carefully made research.
We used our best practices to make sure your new website loads fast. All of the images are compressed to have as little size as possible. Whenever possible we used vector formats - the format made for the web.
Grained is optimized to offer a frictionless experience on every screen. No matter how you combine our sections, they will look good on desktop, tablet, and phone.
Both complex and simple animations are an inseparable element of modern website. We created our animations in a way that can be easily reused, even by Webflow beginners.
Our template is modular, meaning you can combine different sections as well as single elements, like buttons, images, etc. with each other without losing on consistency of the design. Long story short, different elements will always look good together.
On top of being modular, Grained was created using the best Webflow techniques, like: global Color Swatches, reusable classes, symbols and more.
Grained includes a blog, carrers and projects collections that are made on the powerful Webflow CMS. This will let you add new content extremely easily.
Grained Template comes with eCommerce set up, so you can start selling your services straight away.
To give you 100% control over the design, together with Webflow project, you also get the Figma file.